GPT-4 faces a challenger: Can Writer’s finance-focused LLM take the lead in banking?
- We often focus on chatbots built by banks and financial firms, but today, we explore the engines driving chatbot interactions and platform automation.
- Banks typically turn to GPT-4 for LLM solutions, but a potential rival is emerging. San Francisco’s Writer, a gen AI company, is pushing forward in enterprise AI with domain-specific LLMs like Palmyra Fin.
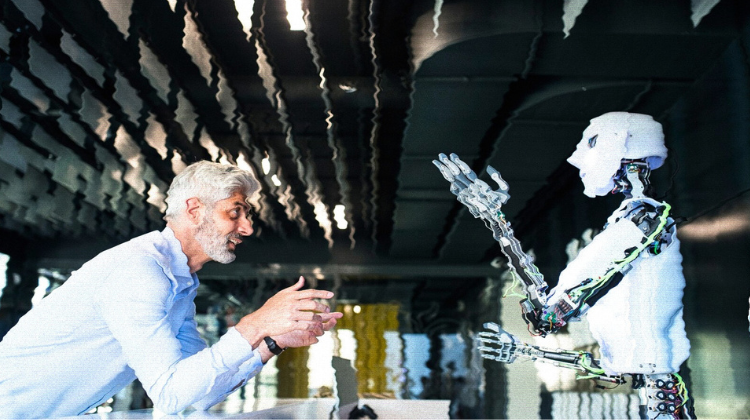
Banks are heavily investing in Large Language Models (LLMs) to enhance both internal operations and customer interactions — yet building a model that excels at both is a significant challenge.
A recent study by Writer, a San Francisco-based generative AI company that provides a full-stack AI platform for enterprise use, found that ‘thinking’ LLMs produce false information in up to 41% of tested cases.
The study evaluated advanced reasoning models in real-world financial scenarios, highlighting the risks such inaccuracies pose to regulated industries like financial services. The research also showed that traditional chat LLMs outperform thinking models in accuracy.
LLMs are used in three main ways within financial services:
- Platforms for operations & automation – LLMs power internal enterprise platforms to streamline workflows, automate document processing, summarize reports, analyze data, and assist employees. For example, Ally Bank’s proprietary AI platform, Ally.ai, uses LLMs to improve its marketing and business processes.
- Task-specific AI assistants – LLMs enhance specific financial tasks such as fraud detection, compliance monitoring, or investment analysis. An example of this is J.P. Morgan’s IndexGPT, which aims to provide AI-driven investment insights.
- Chatbots & virtual assistants – LLMs improve customer-facing chatbots by making them more conversational and executing basic tasks. Bank of America’s virtual assistant, Erica, provides banking insights to its customers.
We often focus on chatbots built by banks and financial firms, but today, we explore the underlying technology behind them — the engines driving chatbot interactions and platform automation.
We take a closer look at the LLMs driving these AI systems, their challenges, and how financial firms can train enterprise-grade models to capitalize on their potential while controlling their risks.
Thinking LLMs vs. traditional chat LLMs
Thinking LLMs, also referred to as CoT (Chain-of-Thought) models, are designed to simulate multi-step reasoning and decision-making processes to provide more nuanced responses beyond only retrieving or summarizing information, says Waseem Alshikh, CTO and co-founder of Writer.
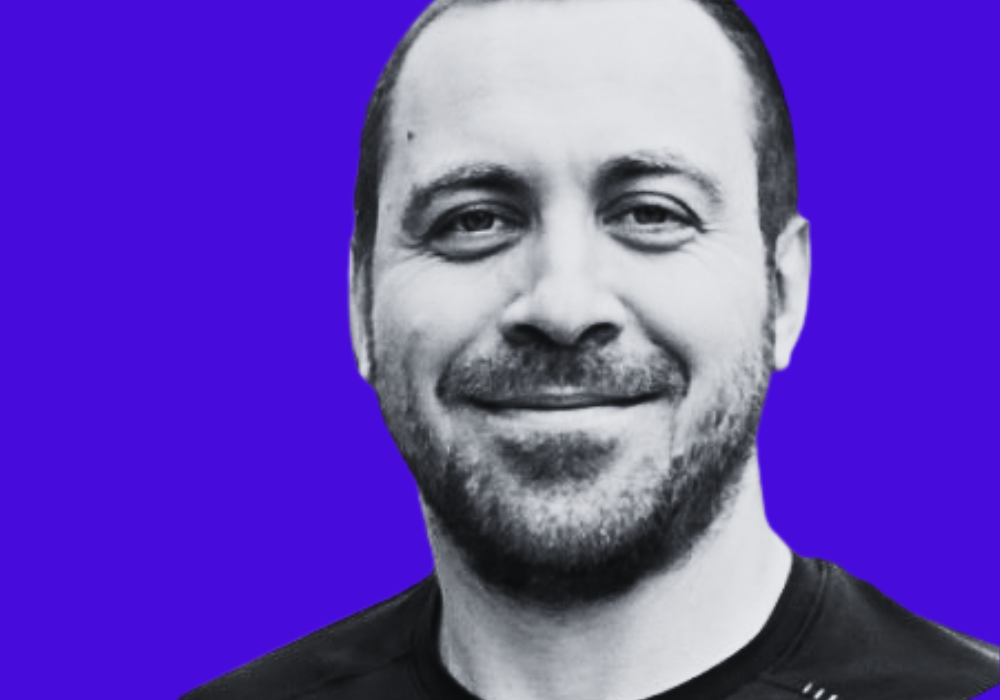
Morgan Stanley’s AI Assistant, for example, uses OpenAI’s GPT-4 to scan 100,000+ research reports and provide quick insights to financial advisors. It enhances portfolio strategy recommendations by summarizing complex data beyond retrieving reports.
“These models are not truly ‘thinking’ but are instead trained to generate outputs that resemble reasoning patterns or decompose complex problems into intermediate reasoning steps,” Waseem notes.
Morgan Stanley’s AI tool encountered accuracy issues stemming from hallucinated responses. Shortly after its launch in 2023, sources within the company described the tool as ‘spotty on accuracy,’ with users frequently receiving responses like “I’m unable to answer your question.”
While Morgan Stanley has been proactive in fine-tuning OpenAI’s GPT-4 model to assist its financial advisors, the company acknowledges the challenges posed by AI hallucinations. To reduce inaccuracies, the bank curated training data and limited prompts to business-related topics.
Traditional chat LLMs, however, tend to be more accurate, according to Waseem. These models mainly use pattern matching and next-token prediction, responding in a conversational manner based on pre-trained knowledge and contextual cues. While these models may struggle with complex queries at times, they produce fewer hallucinations, making them more reliable for regulatory compliance, according to Writer’s research.
Bank of America’s virtual assistant, Erica, uses a traditional chat model to assist customers with banking tasks like balance inquiries, bill payments, and credit report updates. By leveraging structured data and predefined algorithms, it provides accurate and reliable responses while reducing the likelihood of misinformation.
But how can financial firms navigate the trade-off between AI sophistication and accuracy?
Best practices for implementing thinking LLMs in financial services
Given the advanced capabilities of thinking LLMs, financial firms can’t simply rule them out, but they can deploy them effectively with the right strategic approaches.
Waseem outlines the key steps:
…