The Future Unveiled: Generative AI’s influence on financial institutions, from Customer Care to Fraud Prevention
- In finance, generative AI transforms customer service with advanced conversational AI and NLP, boosting satisfaction and revenues.
- Additionally, it impacts fraud detection by crafting synthetic data, cutting false positives and improving detection rates significantly.
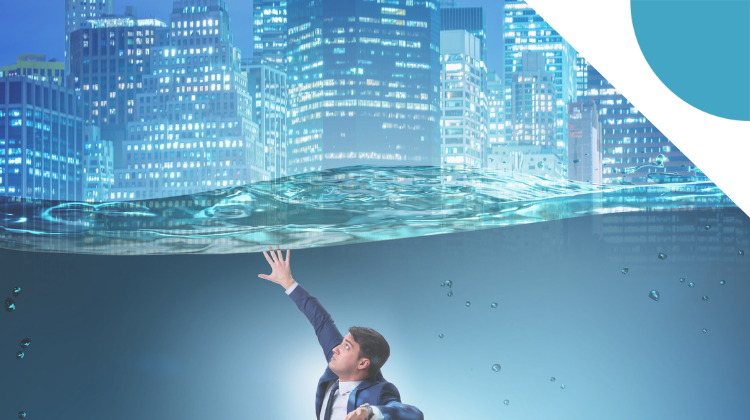
Jacqueline White is President of i2c
Generative Artificial Intelligence (AI) is a hotly debated topic today. There are so many critics and champions of this technology that it is difficult to remain neutral for very long. This is especially true of banks and financial institutions that are constantly seeking ways to drive enhanced accuracy, cost-effectiveness, and profitability through improved decision-making processes and business efficiencies.
In recent months, generative AI programs like ChatGPT for text and MidJourney for image generation have redefined the expectations for AI output. These programs are trained using an extensive collection of documents, enabling them to comprehend questions more effectively. In order to boost profitability, financial services executives cannot afford to ignore some of the benefits of this evolving technology.
One key area where generative AI is making a significant impact is customer service. Customer service plays a pivotal role in driving customer satisfaction, retention, profitability, and lifetime value, with a potential revenue increase of 2% – 7% according to a study by McKinsey. By utilizing chatbots and virtual assistants—augmented with Natural Language Processing (NLP) — companies can simulate sophisticated and more “human-like” conversations in areas like customer onboarding, managing inquiries, advisory services, multilingual support and dispute handling.
These conversational AI solutions offer more enhanced customer support because they integrate seamlessly with other systems and databases. And while many critics claim that NLP systems frequently misinterpret queries and provide incorrect feedback, machine learning (ML) algorithms enhance the reliability of responses over time. As a result, conversational AI may even be used to improve customer service training and streamline sales compliance monitoring.
Another core area that can significantly impact operational efficiency is fraud detection and monitoring. Digital preferences have made payment fraud an area of growing concern in the United States. In 2022, according to Statista, fraud across all payment types amounted to more than $4.5 billion. Card-based fraud is one of the most significant expense lines for banks and financial institutions, with U.S. issuers expected to lose over $165 billion to it in the next ten years.
A superior customer experience strikes a balance between providing access to, and protection of, funds. Because minor improvements in fraud prevention can significantly impact the bottom line, financial institutions have invested in ML models to detect fraudulent transactions. However, these ML models can create false positives (authentic sales classified as fraud and excluded from being recorded as a legitimate sale).
To address the annual loss of approximately $8 billion in sales and to prevent customer attrition caused by declining genuine transactions, the use of advanced generative AI tools can generate high-quality synthetic data. This data can be used to train fraud models, enabling them to effectively differentiate between legitimate and fraudulent transactions. In this way, Danske Bank in Denmark was able to improve detection rates by 50% while reducing declines on account of false positives by 60%.
This technology can also create risk models based on the properties of any data set and the relationships between many complex variables. Existing models are based on financial indicators like savings, income, and assets which are increasingly being viewed as sub-optimal in a digital world. Today, however, individual potential, transactional intent and social networks may be more critical in assessing risk than a thin credit file. This is why generative AI models are ideally suited to meet the needs of a flexible risk management infrastructure, which could make a meaningful difference in at least three critical areas:
- Risk assessment: NLP models allow banks to synthesize and summarize vast quantities of internal and public data to provide human decision makers with actionable insights rather than analysis.
- Credit scoring and loan approvals: Banks can use unstructured data and qualitative factors (like social media activity, online behavior and transaction patterns) to assess a consumer’s ability to repay a loan.
- Regulatory compliance: Generative AI techniques help banks to automate the extraction, analysis and organization of data while minimizing human error.
Generative AI’s time has come. Recognizing the need for it is a crucial first step. However, finding the right partner to support planning, development and execution is critical. This is because the technology raises many questions about the reliability of model output, and the privacy of data sourcing and usage. As these tools evolve, banks and other financial institutions need to work with reliable partners to navigate the ethical, regulatory and operational concerns inherent in this technology to fully leverage its advantages.
Despite these challenges, generative AI’s seemingly human capabilities hold much promise for what the future has to offer. With the potential to revolutionize the financial services industry, AI has the capability to redefine core functionalities such as customer experience and fraud detection, as well as to enhance value-added capabilities like product design, financial planning, and portfolio management.