The Quarterly Review: Current’s CTO Trevor Marshall’s working on building better models and shooting for step function improvements
- CTO of Current Trevor Marshall dives into his focus on optimization and building better tools for his teams.
- We also get a novel look at what success means to a neobank's CTO and summarily, 1% to 5% improvements aren't enough.
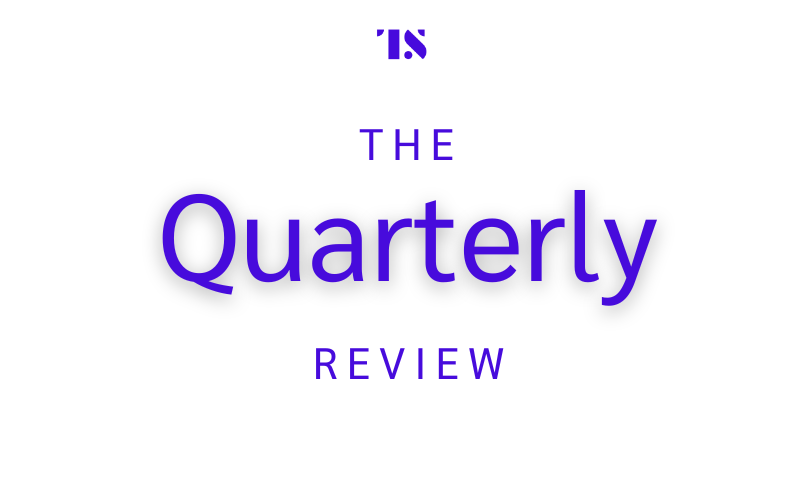
Notes from the desk: Welcome to this month’s Quarterly Review, a series where I dive into what executives from some of the best brands in financial services are focusing on in this quarter, as well as how they are planning to achieve their goals. It’s a chance for the industry to learn about what goes on behind an FI’s four walls and how leadership manages their priorities.
But that’s not all: a review implies no mandates, a check in. So stay tuned next quarter to learn whether the executive achieves his plans and translates theory into reality.
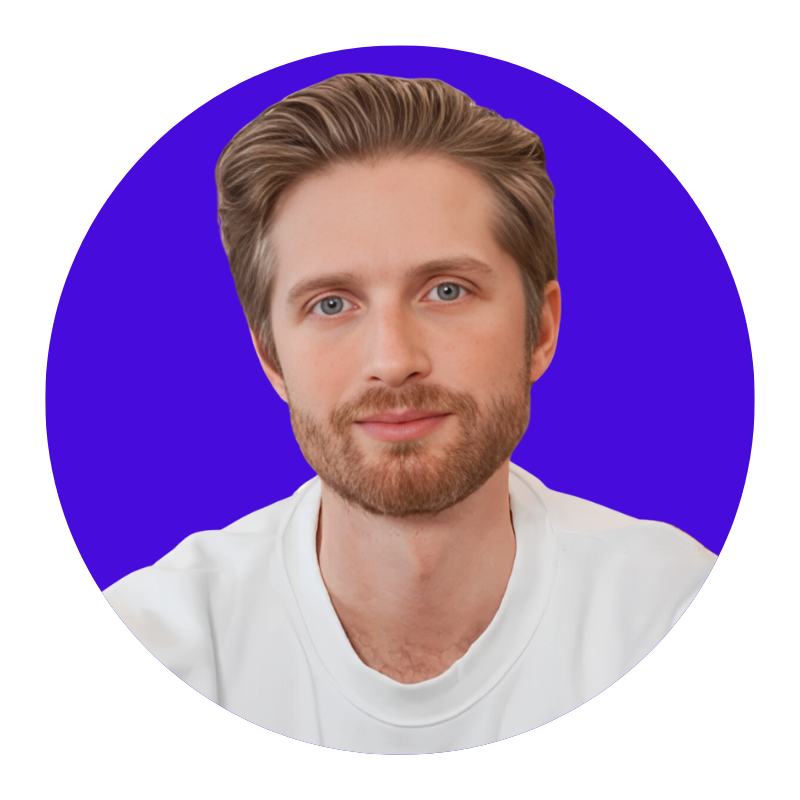
In this edition, we focus on Trevor Marshall, CTO and co-founder of Current.
One company that has steadily blended tech and banking to produce competitive products and customer experiences is Current. With its own core and the launch of two new products in 2023, Current’s CTO Trevor Marshall has a top-down view of the company’s nervous system: its entire technological infrastructure.
Marshall’s to-do list for this quarter is all about optimization and improvements. His metric of success is big noticeable improvements and the ability for his team to focus on explorative tasks rather than spending time doing what can be automated.
The focus: Optimizing existing ML models in risk and growth
Marshall: We are doubling down on the incredible growth we’ve seen over the first half of the year by increasing investment in our data infrastructure and deploying ML models in many parts of the business. This investment will drive greater efficiencies across the board, and enable further scale.
The most successful models we’ve developed include ATO detection, payroll prediction, transaction risk assessment, and delinquency prediction. These models benefit from our advanced data infrastructure, powered by Google’s Vertex AI, which enhances their performance and enables rapid retraining.
Our company is committed to improving financial outcomes for our members, a mission evident in all our products and services. They’re crafted to meet current needs while remaining adaptable to future changes in member requirements. Therefore, our capability to train our models to detect and adapt to evolving behaviors is pivotal to achieving this mission, and it’s one of the largest focus areas we have over this upcoming quarter.
The deployment of these ML models will be in two broad categories:
i) Risk: This is a hugely efficient area for us. Some examples of that are our ability to predict account takeovers, payroll frequency, and potential delinquency.
ii) Growth: On the growth side, there’s a couple models in place, one of which is our ability to predict if someone’s going to put their payroll onto Current. We’re able to predict that with about a 90% precision in the first two days of a user being on our platform.
We’ve seen some pretty amazing improvements already in these areas and so that’s where we’re continuing to focus. Here, having the proper data infrastructure in place is crucial. Because we are a relatively small team, and we have experienced an increase in our growth rate by over 100% in the last year – the only way for us to really keep up with that is to have a data driven approach to managing risk.
Plan of action
As our members’ needs and behaviors evolve, we are revising our data definitions, increasing our rate of feature engineering, and retraining our models accordingly. Here are a few items, I am focusing on:
i) Data infrastructure: We have to keep things like automated periodic retraining to prevent model drift top of mind. We also have to think about deploying our analytical research resources to ensure that we have tight controls around model performance over time. Conduct constant investigation into feature engineering required to improve and release new versions of the models that we have in production.
ii) Better tools: From a CTO’s perspective, I think about supporting initiatives that take a lot of the manual ML Ops work off of people. It’s not a good use of time even for running or retraining a model when all this stuff can be automated. The infrastructure that we have in place currently, ensures that you don’t need to go through the brain damage of reconnecting all of these wires together in order to achieve relatively mechanical activities and outcomes.
So one big area for us is ML Ops automation and making sure that our team understands how that is working, and they can be more focused on the explorative space of feature engineering and identifying new signals to be brought in. We’re leveraging a lot of what Google has on Vertex AI for ML Ops, but we’re layering the business level automations, which would still require analytical resources, on top of that. We’re trying to take that burden off that team and ensure that they’re really focused on a combination of QA, discovery, and feeding that back then into our engineering process.
One of the things that we’re working towards on a few of our models is things like weekly retraining, where the new models are run in a shadow mode. Because of that you can see the performance of the model without it actually going to production. Once it looks good we decide to promote it. That’s a really good milestone — especially once we’re there on at least two of the models that we have in production.
Getting to that place where analysts and data scientists are really focused on thinking and less focused on sort of the mechanics of the system. That’s the big state, and we’re there in some places, and we’re trying to get there everywhere.
iii) Marked improvements: There are a ton of these social scams that are happening, and we’re no exception to that. We recently deployed a new version of our account takeover prediction model, and we were able to drop the account takeovers –specifically the account takeover losses, people who have money stolen from them – by 90%. That’s a step function change. It’s not really like these 1% to 5% improvements. We are asking: where are the step function opportunities? That’s what success is to us. That’s really what we’re targeting right now, because there’s so much opportunity once you have data in the right place.