The story of Erica, Bank of America’s homegrown digital assistant
- Sometimes a bank chooses to build a feature that sets the tone for the industry. Today's story is a case study into such a case, where Bank of America's Hari Gopalkrishnan dives into how the bank developed its digital assistant and how its role has expanded over the years.
- On the show, Hari dives into the challenges the firm faced when building Erica and then takes his sights to the future, giving us a never-before-seen look at how one of the biggest banks in the industry is thinking of Gen AI.
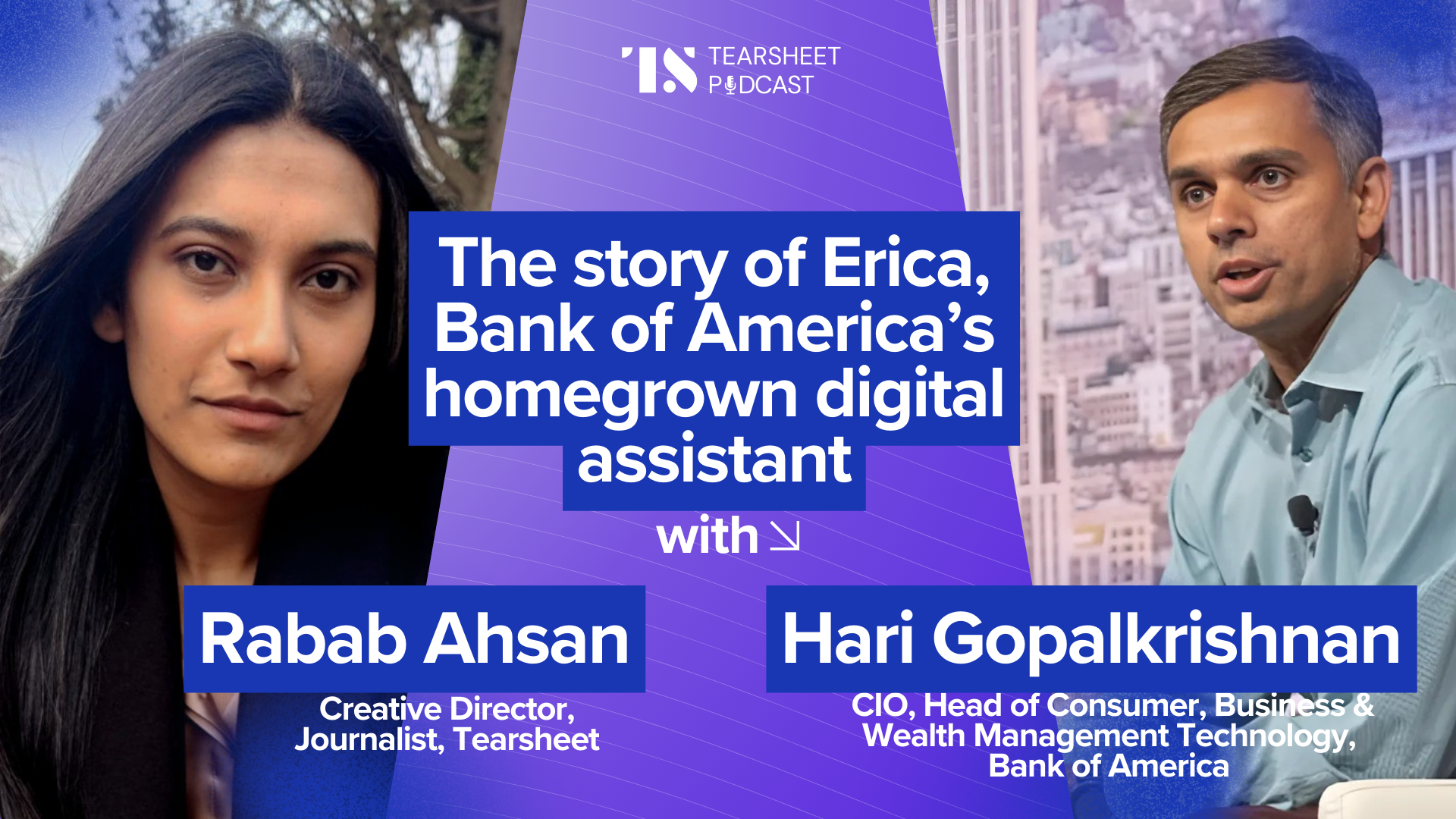
Banking digital assistants may be common now, but in 2017, Bank of America was one of the first to be thinking about how they make the firm’s customer experience more powerful. The answer was an in-house build of a digital assistant that required the firm to hire PhDs in linguistics and build a collaboration structure that could facilitate teams from different departments.
In 2024, BofA clients interacted with Erica 676 million times bringing its total interactions since its launch in 2018 to 2.5 billion.
On the show today, Hari Gopalkrishnan, who leads Bank of America’s Consumer, Business & Wealth Management Technology team, joins us to tell the tale of how the firm built its industry-leading digital assistant, Erica.
Hari shares how the firm has gradually expanded Erica’s remit beyond consumer banking to also include multiple lines of business and individual and corporate clients across the firm’s global footprint.
It’s a dive into what it takes to push the boundaries in this industry, how the firm thought about development, testing, expansion, and how Erica’s capabilities can be expanded with the recent innovations of Gen AI.
Listen to full episode
Subscribe: Apple Podcasts | SoundCloud | Spotify
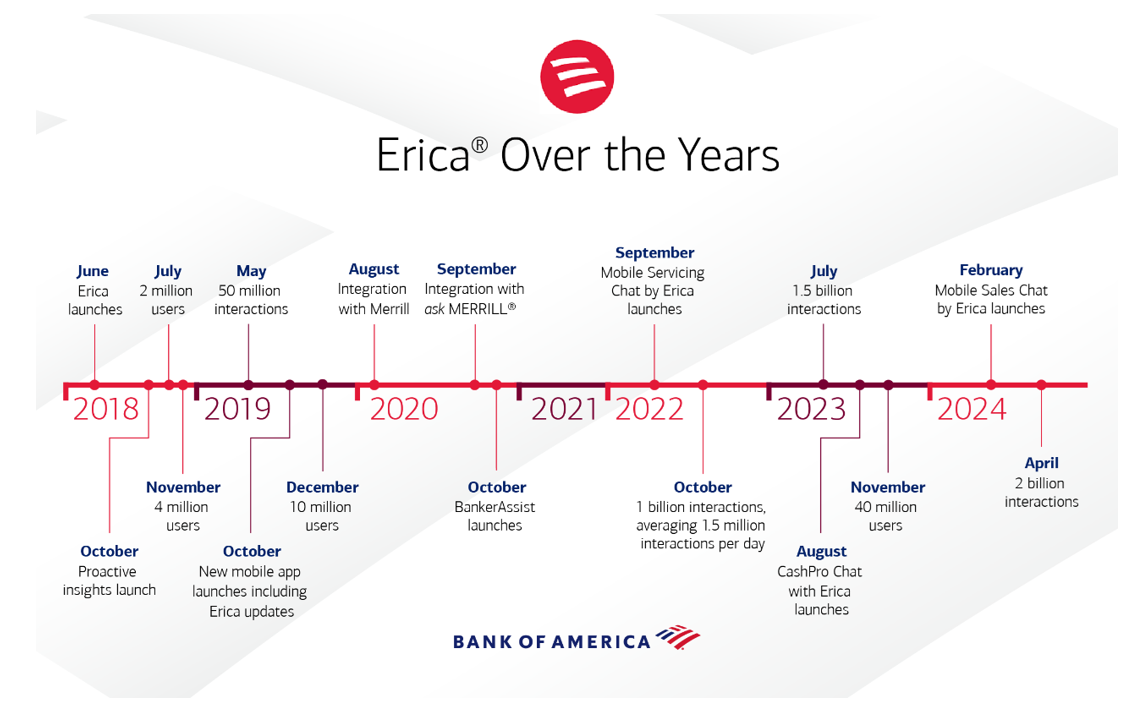
CX as the impetus behind Bank of America’s investment into building Erica
Back in 2017, Hari Gopalkrishnan’s team realized that despite the expanding remit of the BofA app, customers were still flocking to traditional channels like banks and call centers before they tried self-serving. A dissection of this behavior served as the catalyst for the Erica build.
“We were putting tons and tons of features into our app, a five inch screen, and our customers were still walking into the branch, calling into the call center … It’s hunting and pecking, and it was hard to navigate once you put more than 10 or 15 key features. And so our first insight was, you want to be able to have your customers interact with a platform in a way they choose, not the way you choose,” said Hari.
Why Bank of America decided on a DIY approach to build Erica
Although the industry is pretty well-versed in building, deploying, and improving chatbots now, the landscape was quite different in 2017, when BofA had first thought of developing Erica. There was no blueprint for what a banking digital assistant looked like and technology providers were few and far between. The slim pickings in the marketplace, as well as the limited applicability most software had in the financial services, fueled an internal build.
“There were a bunch of startups that were coming out, we spent a few weeks with them and either they disappeared or were going to get gobbled up. So it was a very volatile marketplace for acquiring software that did this. People were trying to play in this game, but weren’t quite getting it. The ones that understood some level of NLU (Natural Language Understanding) didn’t understand financial ontology,” he said.
“We did an assessment, and we looked at third parties, like we always do, but at the end of the day, it just wasn’t going to work out. At the same time, we found a couple of really good open source NLP engines. They were strong, solid, and very well regarded in the industry. We actually hired up a team. We always had good technical engineers. But we also actually hired people with PhDs in linguistics to work on this. Then we started to work with our teams to figure out: what is the digital experience going to look like?”.
How Bank of America structured teams across the org to build Erica
Building Erica required BofA to think across organizational silos, and really invest into creating a collaboration framework that would allow Erica to improve CX without compromising on risk tolerance.
“We actually took the Agile construct to the next level. We had teams set up in [different] regions which were actually in the room. It was the engineering team, the UX team, the appropriate legal team, all opining day in, day out, on all aspects of the platform. The sprints were not just engineers running off and UX coming in weeks later. It had UX teams embedded. In fact, when I used to visit the teams, it was sometimes hard to tell who was in the design team and who was in the engineering team. That was actually the power of how this came together,” Hari shared.
The fork in the road: User testing proved presumptions wrong
Designers and developers have a conceptual map of the software they are building and they also spend time trying to understand their users’ behaviors and pain points. But there is no silver bullet for getting everything right, right off the bat. Hari shares how testing motivated a major pivot in Erica before it launched.
“We thought we would have to invest just as much in voice as in text -– that people would half the time talk into the app, and half the time they would type into the app. Then we go to a small group of customers, and we get more feedback from this. And the feedback we got there was people were just typing in text 90% of the time. They were rarely using voice.”
Gen AI potentialities for Erica
Bank of America is not sleeping on Gen AI – it’s just chosen to stay quieter than most. “As we look at the emergence of Generative AI, we actually see that classification can actually get a lot better. You can actually talk even more naturally in a natural language. So that is just a natural sort of expansion of where we go with Erica. We have about 25 different proof of concepts right now, many of them are actually about to get into production, which use a Large Language Model in some way, shape or form, to continue to enhance the work that we’ve been doing,”.
—
The following excerpts were edited for clarity
BofA’s blueprint for Erica’s expansion into multiple lines of business
Some of it actually is using fit for purpose language models that are pre-trained on certain things. So, for example, for employees there are available technologies that actually are trained on things like integration into your HR system and integrating into your help desk system. We don’t want to go build a whole bunch of things that actually have been built by somebody before.
The reason we built what we built is because nobody was building that before. When it came to employees, we realized that we can leverage all the goodness we have on NLU (Natural Language Understanding) and User Experience. We also found that there are available models that actually do a really good job of NLU to service intent and to calling of existing HR platforms.
We had to figure out case by case, do we start a native build? Do we integrate with existing models when it comes to Erica for business banking there? We had to go to a different set of data sources. You had to make sure those sources were clean. You had to make sure, in some cases, that there was an API available to make that interaction happen … in some cases, many of the services in the past may have been built for a specific User Experience or a specific application, we had to make sure that they get rebuilt or reimagined to be invoked by a chat bot, because sometimes you may need clarifying steps. You may have a multi-step process before you actually call an interaction. That also helped us become better in our core platforms, because that helps us now be ready for the future.
How BofA is balancing ROI, risk, and innovation when it comes to Gen AI
We have an AI Council. Even though we’re obviously a very large company, we try to work in a very integrated fashion and look to learn from everything that’s going on across the company. There are lots of parts of this company, and we come together. You could say it slows us down, but we’re okay with that. We ask, what are the pilots and POCs you want to run? And why do we want to run those POCs and pilots? The people involved in that council involve senior business leaders, senior strategy leaders, senior risk leaders. We’re asking, does this thing align with our risk framework? We have a risk framework that has 16 points of risk. You can imagine bias, intellectual property, transparency, and explainability, in there.
Is the work you’re going to do, going to abide by those risk frameworks? Is there an adequate human in the loop so that you can make sure that the thing doesn’t run away? How do you measure the performance? What guardrails are you going to implement? Those are the things we look at as we implement any of these proof of concepts and eventually take them to commercial use.
The second part is we also look at, what is the mindset you have on the ROI generation of doing this work, because none of this stuff is cheap. This is something everybody is wrestling with. There’s so much hype out there that people are throwing out, I’m going to spend a billion dollars. I’m going to spend $5 billion and when you ask the question, tell me what your bottom line is going to be, what are you going to get in return? The answers are a little bit more diffused.
So we’re taking an approach of saying, we want to understand how work gets done. We want to understand activities, jobs, tasks. We want to understand what part of those tasks cost, what money. And then, when you implement solutions like this, what’s the ROI?