A deep dive into how Amex’s new Frontier Research Team is using AI and ML to build better modeling solutions
- In this deep dive, we explore the role of Amex’s newly formed Frontier Research Team — where it sits within the organization and how it helps surface emerging AI use cases.
- We also look at the team’s learning culture, and the tools and resources that empower its data scientists to stay sharp, inquisitive, and continuously evolving.
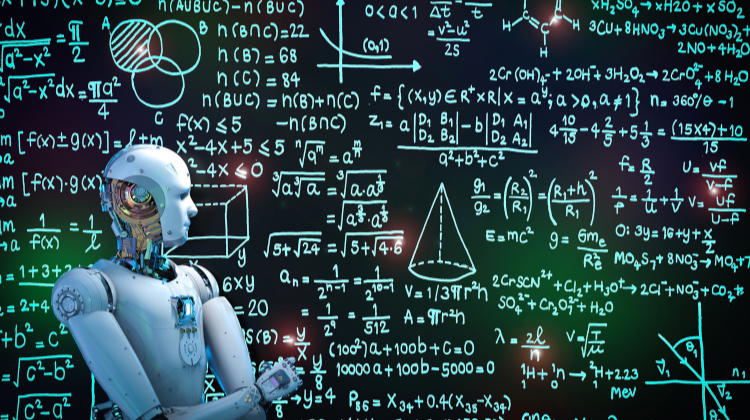
AI has found one of its strongest footholds in financial services through payments – and payments firms are beginning to lean in hard.
American Express (Amex) is one of the legacy payments players tapping into machine learning (ML) and artificial intelligence (AI) to improve how it handles risk and credit decisions. The company is also actively exploring the tech’s potential, with a newly created team focused on uncovering new AI/ML use cases.
The Frontier Research Team is tasked with pushing the boundaries of what AI can do. In this deep dive, we explore the Frontier Research Team’s role within the company structure and how it surfaces new use cases. We also look at the learning culture, tools, and resources that help its data scientists stay ahead of the curve, continually learn, and level up.
Where Frontier Research anchors itself in Amex’s vision
To better understand the role of the Frontier Research team, we first look at the structure of Amex’s Credit and Fraud Risk division, offering context for how the team fits into the broader risk strategy.
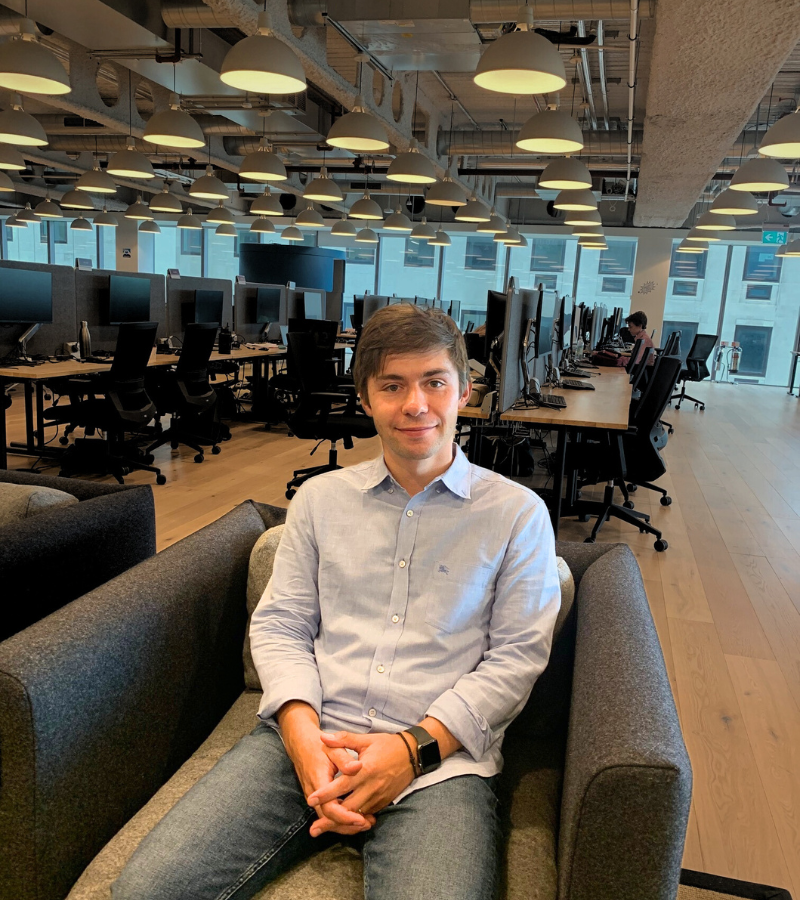
Anton Hinel, VP of Frontier AI Research and Customer Insights at American Express, explains that the Amex Credit and Fraud Risk (CFR) division is in charge of providing Amex with the data and tools to make the right credit decisions to mitigate scams and fraud.
“We’re a global team with colleagues in 17 markets, including centres of excellence in Singapore and India that focus on decision science,” says Hinel.
Within CFR, Global Decision Science (GDS) is tasked with creating and maintaining modelling solutions for credit, fraud, and AI innovation.
Many teams within GDS are responsible for looking after a set of business-specific use cases, such as looking after solutions that prevent fraud. They are responsible for building and monitoring the performance of production models that serve that specific business need.
“Using ML, we monitor transactions and return billions of credit and fraud risk decisions in real time,” he says. “Data scientists within GDS build and maintain these models in partnership with teams across Amex, ensuring the best customer outcomes.”
GDS comprises PhD-level researchers who build ML models, create new big data capabilities, and research ways to resolve issues with existing methodologies.
In addition to their credit and fraud risk objectives, these teams can also create models for marketing to help surface the right offers and benefits for different customer segments.
The Frontier Research Team’s role: The Frontier Research Team is a part of Global Decision Science.
“We were officially formed last year to support a longer-term research and exploration agenda,” shares Hinel. “Prior to the creation of our team, the research agenda tended to be primarily focused on resolving urgent business needs, which is, of course, critical and continues to be done across American Express.”
“But, the idea behind Frontier Research is to give researchers time and space to work on more long-term research — something that is not always possible for a conventional modelling team that owns and maintains a production model, as they typically face tight timelines and a lot of day-to-day responsibilities.”
At a macro level, researchers in the Frontier Research team have two main objectives:
- Identify and investigate methodologies that can be transformational in the way CFR makes decisions and serves its customers.
- Deliver constant learning to enhance enterprise-wide knowledge, publish industry-wide research, drive methodological innovations, and pave the way for implementation.
In terms of structure, the Frontier Research comprises “seasoned professionals, PhDs, and world-class ML researchers. Right now, we have team members across the US, UK, and India focused on developing next-gen modeling solutions,” he shares.
What sets the Frontier Research team apart from GDS is that their focus is on research and the discovery of new knowledge.
“Our goal is to ask the tough questions that hopefully yield answers that apply to the entire company,” says Hinel.
From Concept to Discovery: How the Frontier Research Team hunts for its next big use case
Anton highlights that the Frontier Team’s research is not limited to a specific business use case – but it looks across the entire customer journey within Amex. This can be broadly categorized into three areas:
- Attracting and approving new customers
- Managing their accounts
- Long-term customer experience and payments
“Our process around prioritizing our research ideas is: we focus on trying to resolve problems that are scalable – spanning multiple use-cases and modelling applications,” he notes. “This way, if research turns out to be successful, we can make an impact on the business.”
The team also focuses on enhancing the efficiency of internal operations. This spans a broad array of use cases, including helping strategy team partners streamline daily portfolio monitoring, discovering ways to improve variables for models, and accelerating the time-consuming, manual tasks involved in pre-processing input features.
Case study: A recent example of the Frontier Team’s work involves exploring new ways to pre-process data, to build more effective models for credit decisioning and fraud detection.
“The automated data pre-processing method (something we co-published with Imperial University) is a good illustration of a problem that spans multiple models and applications,” notes Hinel.
In this example, the Frontier team addressed the challenge of feeding complex financial time series data into neural networks, cutting down on the time spent on manual data pre-processing.
When it comes to credit risk and fraud, the data isn’t always straightforward. Irregularities can complicate data processing, such as:
- Transaction Patterns: Data often exhibits multiple distinct behaviours, leading to a multi-modal distribution.
- Credit Utilization: Credit usage is unevenly distributed, which creates skewed data.
- Unusual Spending: Outliers arise from atypical spending behaviours.
- Missing Values: Many customer characteristics used in modelling have a significant proportion of missing data.
- Time-varying Characteristics: Customer characteristics change over time due to macroeconomic shifts, making time series features non-stationary.
Effective data pre-processing enhances training efficiency, allowing for faster iterations and improved model performance. But if data irregularities are left unaddressed, the model may take an excessively long time to train or fail to converge altogether. This can lead to significant performance degradation and statistically biased predictions on out-of-time samples, particularly when using neural networks.
“Our method reduces manual pre-processing effort, improves model performance and training efficiency, adapts automatically to new data patterns, and integrates seamlessly with existing neural network architectures,” he says. “We incorporated best practices into our models, and we still believe the research has potential for further exploration, such as improving the handling of missing data or expanding applications within risk assessment.”
By following this approach, the Frontier Team brings new research to the production teams, who take on the task of building and scaling, which requires extensive testing and iteration.
The journey from brainstorming to prototype to application: At a broad level, the Frontier Team starts by identifying a concept or method that looks promising, then develops an initial pilot using data that reflects possible production use cases.
“This step helps make the idea more tangible and clarifies its application,” notes Anton.
If the results are favorable, the Frontier Team engages with the relevant stakeholders — marketing, fraud, IT, or other internal teams — walking them through the process, collecting feedback, iterating, and partnering to create production-oriented experiments.
“An ideal outcome is when we can graduate a pilot to a team that will then build, scale, and maintain it,” says Anton. But many experiments do not make it to production and fail for one reason or another. “We do not see this as a negative outcome – we see it as a learning that informs our future research,” he adds.
“We also share our findings with our partners [internal Amex teams], so they don’t duplicate efforts and repeat the same attempts in the future.”
How the Frontier Research Team supports its data scientists in staying sharp in AI/ML
A key responsibility of the Frontier Team is staying up to date with emerging AI and ML knowledge and concepts. Equally important is the ongoing development of its data scientists’ skills.
With that in mind, what training, tools, or initiatives contribute to the continuous learning of their data scientists?
Hinel says one key strategy is returning to academia, where teaching and building academic partnerships facilitate mutual learning.
“This is one of the more enjoyable parts of my role because we have the privilege of working not only within Amex but also soliciting opinions and information from outside experts,” he notes. “We have a long-standing relationship with many academic institutions – Cornell University and Imperial College London are a few of those examples.”
The co-authored research paper with Imperial College is just one aspect of that collaboration, explains Hinel. The Frontier Research Team works closely with Imperial’s Department of Mathematics, where it supervises a master’s student on their final-year project. The Frontier Team’s efforts are focused on identifying key technical challenges that could significantly enhance their work in risk assessment and fraud detection.
“This keeps us updated on the latest academic advancements, while also helping find new talent,” he says. He adds that as a result of this partnership, the Frontier Research Team hired one of their newest members, who was the student they collaborated with on the data pre-processing project.
Academic partnerships also enable the Frontier Team to publish external research and participate in conferences, where they get the opportunity to stay updated on the latest advancements in the field.
Internally, the team organizes paper and research reading sessions, with Anton encouraging team members to experiment with public datasets. In his view, platforms like Kaggle, which are popular among data scientists and ML experts for predictive modeling competitions, offer valuable insights into how top researchers solve practical problems.
“They also provide opportunities for the Frontier Team to test new methods and explore research questions,” notes Hinel. “The last Kaggle we sponsored focused on credit default prediction, and it drew about 10,000 participants.”
“As important as research is, without hands-on experience, we wouldn’t know how these concepts and techniques would behave in real-world scenarios.”