The Banking AI Adoption Gap: Leaders pull ahead while others struggle to catch up
- The AI race in banking has clear frontrunners accelerating innovation at twice the speed of competitors.
- Evident co-CEO Alexandra Mousavizadeh unpacks this divide and shares strategies for closing the gap.
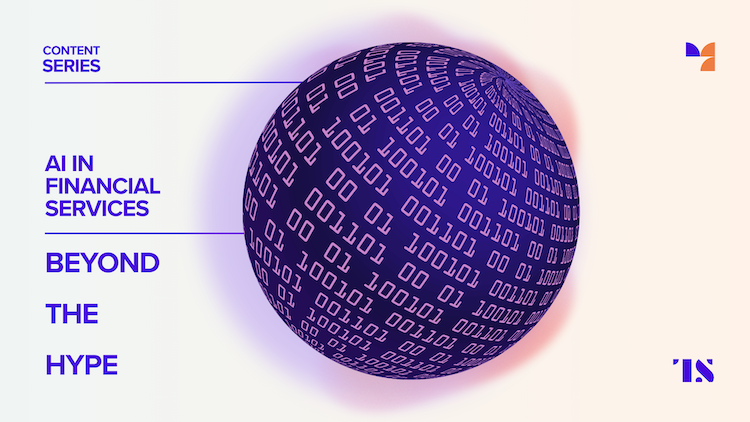
In the push to adopt artificial intelligence, a significant gap is emerging between leading banks and their mid-tier counterparts. Recent research by Evident reveals that top institutions are accelerating their AI maturity at twice the pace of others, creating what could become an insurmountable competitive advantage.
“The leading banks are accelerating like 2x ahead of others, and that just is picking up. The faster they’re going, the faster they go,” explains Alexandra Mousavizadeh, co-founder and co-CEO of Evident.
This widening divide raises critical questions for financial institutions still finding their footing in the AI landscape. What’s driving this gap, and how can mid-tier banks avoid being left behind?
The groundwork advantage
The research shows that leading banks have a head start not just in deploying AI solutions but in laying essential groundwork that enables faster innovation.
“The leading banks are in a position where they have done a lot of the groundwork,” Mousavizadeh notes. “They’ve really looked at their data infrastructure, their cloud strategy, their data strategy, their governance strategy.”
This preparation allows top banks to move quickly when new technologies emerge. When generative AI appeared on the scene, these institutions were primed to integrate it immediately into their existing AI frameworks.
Key takeaway: The foundation matters. Banks that invested early in proper data infrastructure and governance can more readily adopt new AI innovations.
The positive flywheel effect
Top banks are experiencing a self-reinforcing cycle of AI advancement:
- They test new tools more quickly
- Get them into production faster
- Assess impact and ROI more efficiently
- Make stronger cases for additional investment
This creates what Alexandra calls a “positive flywheel” that accelerates their lead over competitors.
Many leading institutions are already moving beyond basic generative AI into more sophisticated applications:
- Agentic AI use cases are in full development
- Some are implementing quantum-inspired algorithms
- Advanced applications include optimizing Monte Carlo simulations, derivative trading, and portfolio optimization
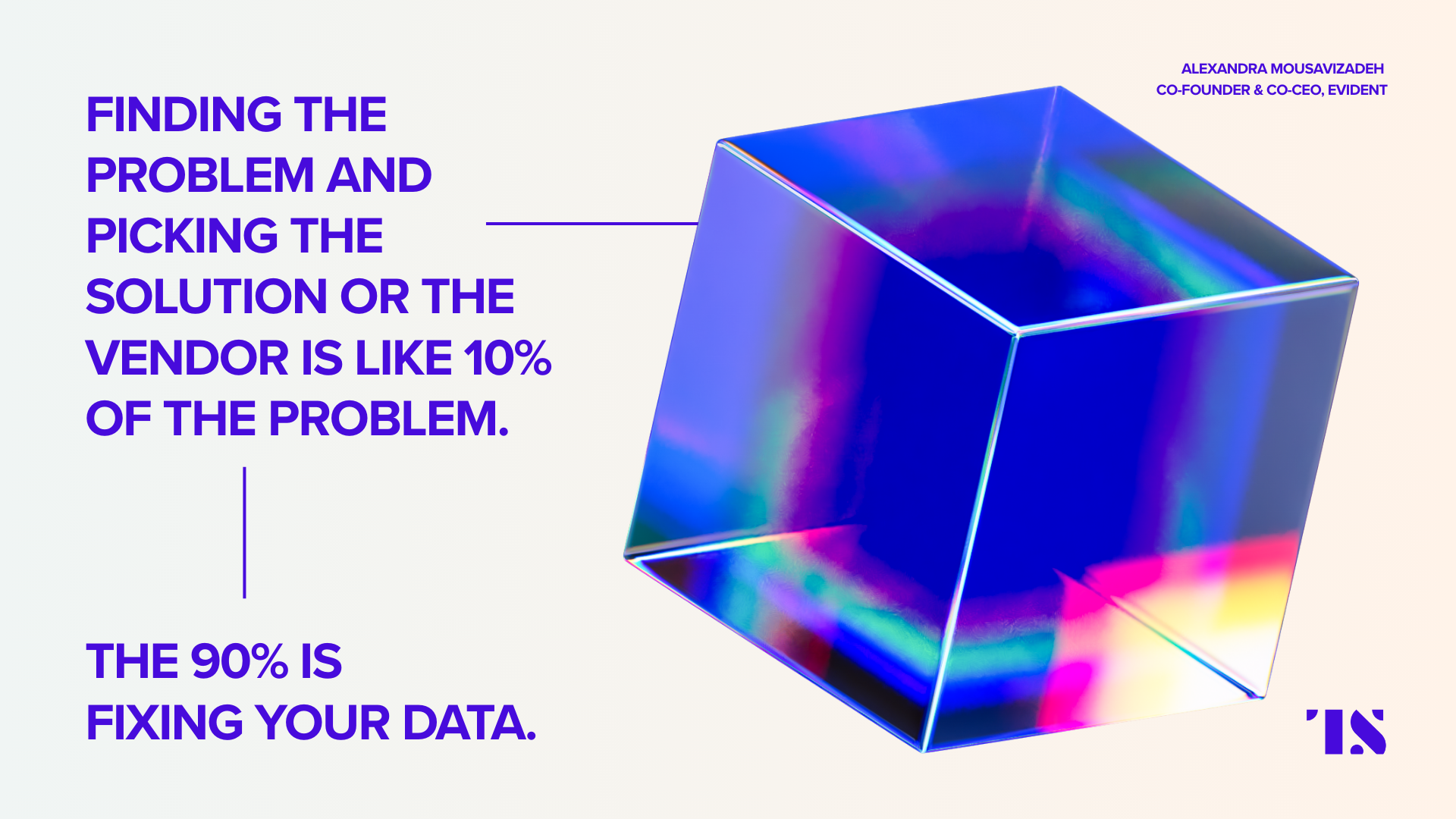
The fast follower challenge
For mid-tier banks attempting to close the gap, there are benefits to being a fast follower. The research suggests these institutions can learn from pioneers’ successes and failures without investing as heavily in trial and error.
However, Mousavizadeh points out a crucial reality: “Finding the problem and picking the solution or the vendor is like 10% of the problem. The 90% is fixing your data.”
This data foundation challenge remains regardless of whether a bank is leading or following, and it can’t be shortcutted.
Key takeaway: Mid-tier banks should focus on getting their data house in order rather than chasing the latest AI tools without the infrastructure to support them.
Cultural resistance: The hidden barrier
Beyond technological challenges, the research uncovered significant cultural hurdles to AI adoption:
“There’s a lot of human resistance,” Mousavizadeh explains. “In the engine room of the banks that are running AI agents… it bypasses some processes that have been manual, and that’s great, but in some lines of business, they’re like ‘no, we don’t want to do that, it’s fine as it is.'”
Successful banks are addressing this through:
- Clear messaging from leadership making AI adoption a priority
- “Two in a box” implementation teams pairing line-of-business heads with AI experts
- Tying AI implementation to performance KPIs
Key takeaway: Leadership commitment is essential. Banks that make AI adoption a measurable priority from the top down show better results than those with scattered, bottom-up approaches.
The ROI measurement challenge
One of the most revealing findings from Evident’s research is the industry-wide struggle to quantify AI’s financial impact. While banks eagerly discuss their AI initiatives, many become “squirrely” when asked about ROI.
“It’s very hard to measure,” Mousavizadeh acknowledges. “The banks that have spent a lot of time and effort on figuring out how to measure impact in a consistent way… it takes an enormous amount of work.”
According to the research, only two banks in their index—JP Morgan and DBS—publicly report comprehensive AI ROI metrics. This transparency comes with both advantages and challenges:
“If you’re making it public that you’ve got 400 use cases in production and your ROI on those is $2 billion… you’ve got to be ready for not only the regulators to say, ‘Let me come and look at those 400 use cases,’ but also at every earnings call, you’re going to be asked about the $2 billion.”
The measurement difficulties center on:
- Attributing costs accurately
- Balancing various metrics (revenue uplift, efficiency gains, NPS scores, integration readiness)
- Maintaining consistent measurement methodologies
Key takeaway: Banks should develop robust ROI measurement frameworks now, even if imperfect, to guide investment decisions and prepare for future regulatory scrutiny.
US vs. European approaches: A study in contrasts
The research highlights striking differences in how US and European banks approach AI development and talent acquisition:
US banks tend toward:
- Centralized AI strategies
- “Tech company” mindsets
- Centers of excellence and AI research labs
- Patent development and academic publishing
- Building solutions in-house
European banks typically favor:
- Federated, bottom-up approaches
- Applied AI research within lines of business
- Partnerships with external vendors
- Buy-versus-build mentalities
These structural differences directly impact talent acquisition. Top AI professionals often prefer environments where they can publish research, participate in academic conferences, contribute to open source projects, and develop patents—opportunities more common in US banks’ centralized models.
“Really great AI talent can choose from a lot of things,” Mousavizadeh notes. “They would like to go to a bank where they can publish research… participate in open source… have hackathons.”
Key takeaway: Banks should consider how their organizational structure affects their ability to attract elite AI talent, particularly as generative AI requires more specialized in-house expertise.
The evolution to agentic AI
While much industry discussion focuses on generative AI adoption, the research suggests some banks may “leapfrog” directly to agentic AI applications.
“There’s almost this [realization that] it’s much easier to actually put agentic AI use cases into production than generative AI,” Mousavizadeh observes. “We’ve always thought about it as you get generative AI sorted, and then you go on to agentic AI, and then probably aspects of that are not quite true.”
This suggests mid-tier banks might find unexpected opportunities to narrow the gap by strategically focusing on specific agentic AI applications.
Responsible AI: Beyond principles to practice
The research found a tripling of banks publishing responsible AI principles, indicating growing awareness of ethical concerns. However, Alexandra takes a nuanced view of this trend:
“One thing is publishing principles. Another thing is how good is your governance and your model risk management—not the same thing.”
She suggests that financial services already provide a robust blueprint for responsible AI:
“Financial services provide a blueprint for how AI should be managed everywhere… You’ve got extreme scrutiny on any dataset… You’re testing your outcomes… Anything before it goes out has a six-month space where it’s really tested and tested.”
Key takeaway: Banks should focus less on publishing principles and more on implementing robust governance frameworks—the industry’s existing risk management approach already provides a strong foundation.
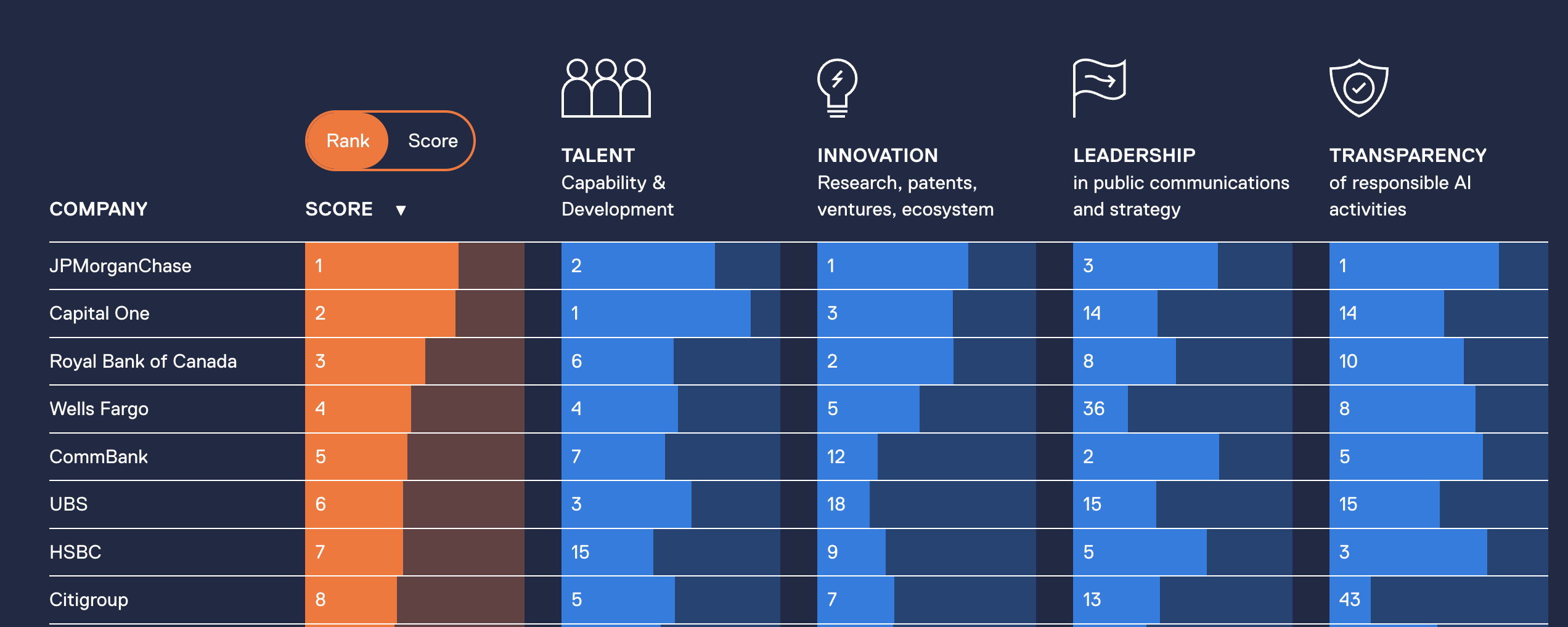
The path forward for banks to adopt AI
For financial institutions looking to close the AI adoption gap, Evident’s research suggests several priority actions:
- Invest in data infrastructure before chasing the latest AI applications
- Establish clear leadership commitment with measurable AI adoption targets
- Develop practical ROI measurement frameworks, acknowledging their imperfections
- Consider organizational structures that attract elite AI talent
- Look for strategic opportunities in agentic AI, not just generative
- Build on existing risk management frameworks for responsible AI governance
The research makes clear that the gap between leaders and laggards is widening. For mid-tier banks, the time for incremental approaches has passed—bold, structured investment in both technology and culture change is now essential to remain competitive.
As Alexandra concludes: “Of course, you’re spending on it, and of course, it’s going to be transformative. Is this going to take a bit? Yes.”