Grasshopper Bank’s Approach to AI: A case study in navigating AI with care
- In an era where companies are quickly embracing AI, Grasshopper Bank is integrating it thoughtfully, emphasizing accuracy over speed.
- With a particular emphasis on generative AI, Grasshopper is applying AI in areas where it can add tangible value without replacing human judgment. We break down how.
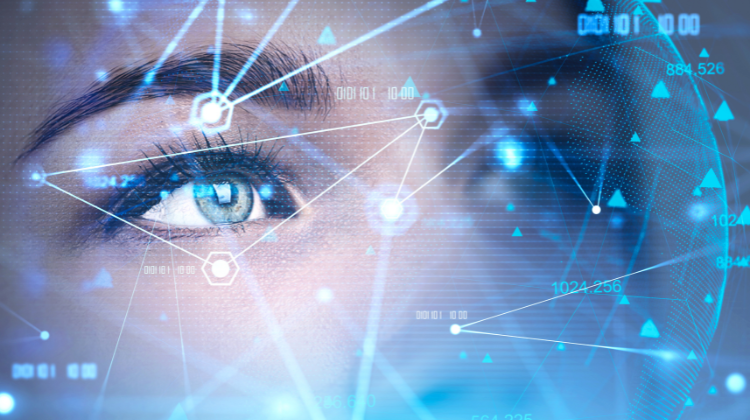
At a time when companies are racing to adopt artificial intelligence, Grasshopper Bank is integrating it thoughtfully, opting for precision over speed.
Instead of jumping headfirst into AI-driven decision-making processes, the bank is making measured and incremental investments in AI, concentrating on its potential to boost productivity, improve operational efficiency, and strengthen compliance.
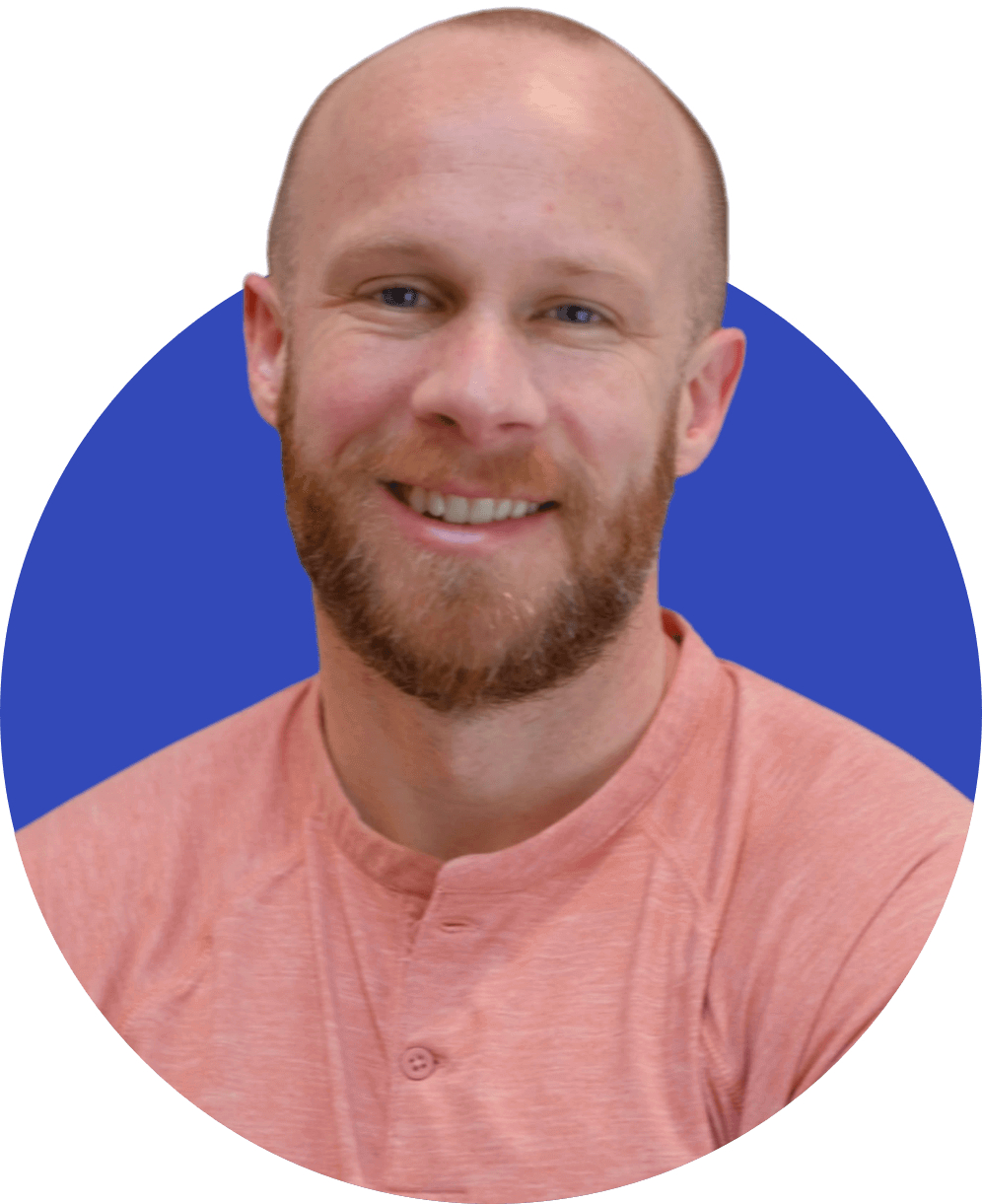
“AI means different things to different people,” says Pete Chapman, Chief Technology Officer at Grasshopper, which offers digital business banking. “We focus on being intentional about how and where we use AI technologies.” With a particular emphasis on generative AI, Grasshopper is applying AI in areas where it can add tangible value without replacing human judgment.
In an interview with Grasshopper’s Chief Technology Officer, Pete Chapman, we learn how this philosophy plays out in the bank’s AI strategy across its business lines.
Grasshopper Bank has 3 main business lines:
- Business Banking for startups and small businesses.
- Lending Solutions for businesses, particularly those in early stages or in need of capital for expansion.
- Banking-as-a-Service (BaaS) and other embedded finance services, such as embedded payments and lending, for fintechs and businesses looking to integrate banking features directly into their platforms.
Grasshopper Bank’s AI vision, split three ways
Chapman explains that Grasshopper’s current AI initiatives fall into 3 primary categories:
1. General Back Office Productivity: Reducing the cognitive load
Grasshopper’s use of AI starts in its back office, where productivity improvements are a priority. The company has deeply integrated Google Gemini into its suite of tools, including Google Workspace, to save knowledge workers 30 hours per month by the end of the year. This includes everything from summarizing emails, generating reports, creating presentations, and automating meeting follow-ups.
“We’re aggressive adopters of new Gemini capabilities and are running structured training and support programs to ensure our employees use the tools effectively,” Chapman notes.
The aim here is not to replace people, but to reduce the time spent on administrative tasks, allowing employees to focus on more value-driven work.
2. Multi-System Process Automation
Grasshopper uses Robotic Process Automation (RPA) to handle repetitive, rules-based tasks across non-integrated systems. These bots significantly reduce manual workloads and help to improve accuracy.
Chapman shares that in the second half of this year, the bank plans to augment these bots with more intelligent AI agents capable of handling broader, more nuanced tasks, creating a foundation for scalable automation across departments.
3. Development and Citizen Engineering: Extending capabilities beyond engineers
AI is also making its way into the development processes at Grasshopper. Developers use ChatGPT and Google Gemini to assist with coding, testing, and documentation, boosting velocity, and improving quality.
However, the company is also laying the groundwork for a Citizen Development program, enabling trained non-engineers to use AI-assisted platforms to build lightweight, non-critical tools.
“This program will be governed under our Software Development Lifecycle and subject to strict oversight from our Infosec team,” says Chapman.
This measure ensures wider access to development tools without affecting the company’s Software Development Lifecycle.
AI in Lending, Risk, and Compliance: Where transparency is key
In areas such as lending, risk, and compliance, Grasshopper applies AI selectively to maintain transparency and uphold regulatory accountability:
Lending and Credit Efficiencies: Working with strategic partners, Grasshopper uses generative AI to streamline portfolio management, with a current focus on annual reviews and portfolio trend monitoring.
While the company has plans to extend AI into areas like origination support, such as drafting credit memos and assisting with pre-decision analysis, final decisions will always be made by human underwriters, notes Chapman.
Enhanced Due Diligence: Grasshopper’s compliance teams use generative AI to assist in Enhanced Due Diligence (EDD) for clients flagged as high-risk. This has helped speed up internal reviews and reduce documentation requests, making the process smoother for both the client and the bank.
Risk Decisioning Model: For now, Grasshopper steers clear of using generative AI in automated credit and risk decisioning.
“We’ve taken this position deliberately,” asserts Chapman. “Modern generative models often function as ‘black boxes,’ and we are committed to explainability and auditability in all client-facing decisions.”
But it’s not a hard no. While that’s the case for now, Chapman says Grasshopper’s stance on generative AI could change as familiarity, comfort, and experience with the tech grow over time.
Guardrails and Governance: Ensuring responsible AI use
To maintain responsible and governed AI practices, Grasshopper Bank blends key strategies with cross-team collaboration for comprehensive oversight:
- Grasshopper isn’t pursuing full AI control in decisioning areas; instead, “we aim for a thoughtful balance,” notes Chapman. “That means automating wherever possible without sacrificing human judgment where it matters most.”
- Human-in-the-loop decisioning is standard across critical processes. AI assists with analysis and recommendations, but humans retain authority over final decisions, particularly in risk, lending, and payments.
- Escalation triggers are built into internal systems. If a transaction or loan application presents uncertainty or flags certain risk markers, it is automatically routed to a human for review.
- Grasshopper also has a cross-functional AI Working Group that meets weekly to identify inefficiencies and champion new use cases. This team includes representatives from Risk, Lending, Ops, Technology, and Compliance, among other business lines. They operate with a defined “hours saved” target and manage a 9 – 12 month AI roadmap, similar to a product roadmap.
- An AI oversight team within Infosec and Technology evaluates tools and reviews models, with all findings reported to the Board’s Tech Committee.
Learning from Mistakes: A moving target
Even with solid strategies and coordinated efforts from both AI-specific and cross-functional teams to plan, implement, and monitor AI, errors can still occur. No AI system is perfect, and Grasshopper is no stranger to challenges brought on by the tech.
Chapman acknowledges that mistakes are inevitable, and he believes it’s your response to them that decides whether you stumble or grow from the experience.
“Rather than viewing these misclassifications as failures, we treat them as opportunities to continuously improve our systems,” he says.
Chapman reflects on instances where AI has made incorrect risk assessments — flagging a customer or transaction as high risk when it was actually low, or vice versa — and missing important alerts or triggering false ones that wouldn’t have been flagged in a human review.
When such incidents occur, Chapman explains that the bank’s next course of action is to:
- Escalate the case to a human reviewer — typically a member of Grasshopper’s compliance or fraud team — who performs a quality check (QC) and determines the appropriate resolution.
- Log and document the issue, then conduct a root cause analysis to understand what led to the incorrect rating or alert.
- Refine the model inputs, rules, or thresholds as needed to prevent similar issues in the future. In some cases, this may involve retraining or tuning models or making procedural adjustments.
Grasshopper Bank’s approach to AI is far from seismic, but it’s thoughtful and measured, which is perhaps even more important. The company isn’t hastening to replace human decision-making with AI; instead, it’s leveraging AI as a tool to complement its human workforce capabilities.