‘Banking’ on AI: How four financial leaders are building trust, not just technology
- This article dives deep into four companies' strategies, exploring how each firm is contributing to a broader vision of enhancing financial services through artificial intelligence.
- It’s about how they're running the new tech — and what it takes to actually build AI that earns trust, drives value, and scales.
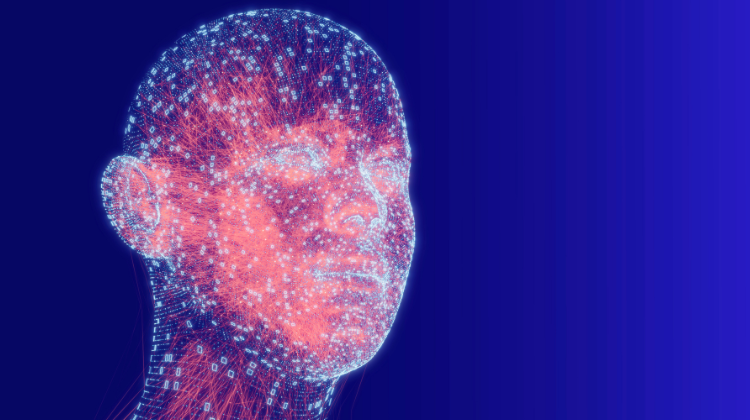
A prominent move is underway in the halls of legacy financial firms and the boardrooms of fintechs as AI nudges a new phase of innovation in financial services. One forged in trial-and-error, feedback loops, backend rewrites, and the messiness of real-world customer problems.
Companies are trying to make sense of the new tech and how to use it, but some have a stronger grasp of their AI roadmap than others. American Express, Grasshopper, Fifth Third Bank, and Credit Karma are four financial firms contending with the impact of AI on their products and customers. Each has its own approach to putting it into action and is at a different stage of AI maturity. With varying degrees of risk, ambition, and technical infrastructure, these firms are converging on a singular goal: making AI work in the real world for their employees and customers.
Amex and Grasshopper are tuning AI to rewire how decisions get made and solutions surface behind the curtain. Fifth Third and Credit Karma, on the other hand, are putting AI on stage — rebuilding their customer experience around it. Think of it as two sides of the same coin: Amex and Grasshopper are strengthening the core, where AI shapes process and possibility, while Fifth Third and Credit Karma are minting a new face for the coin, one that’s more intuitive, responsive, and human.
It’s not about who’s ahead in the AI race. It’s about how they’re running it — and what it takes to actually build AI that earns trust, drives value, and scales.
This article dives deep into these four companies’ strategies, exploring how each firm is contributing to a broader vision of enhancing financial services through artificial intelligence.
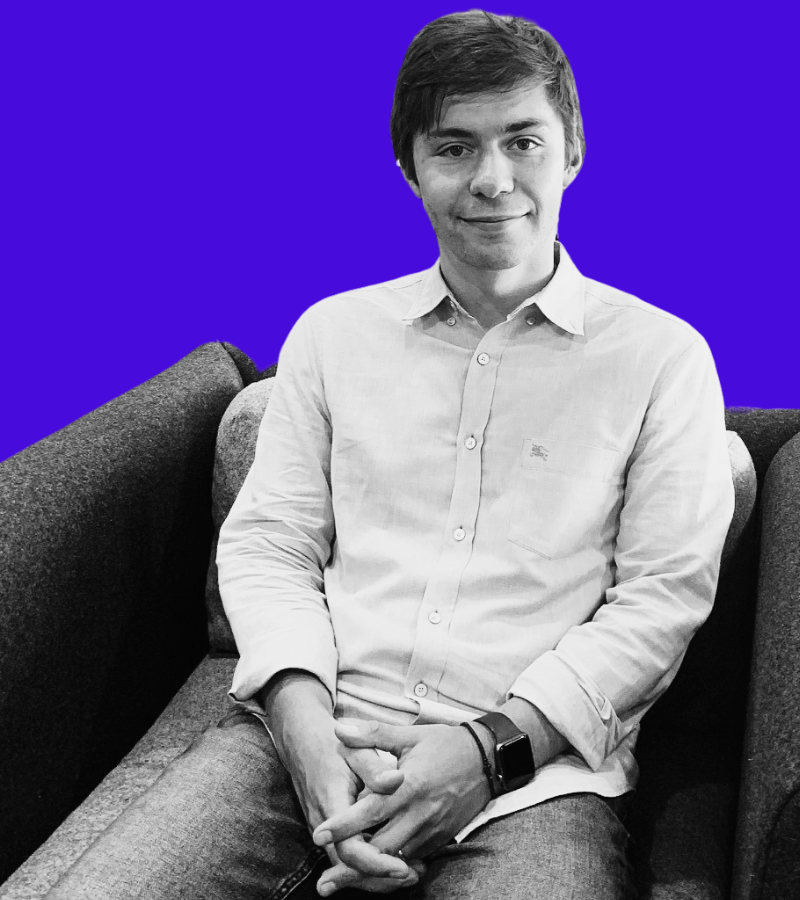
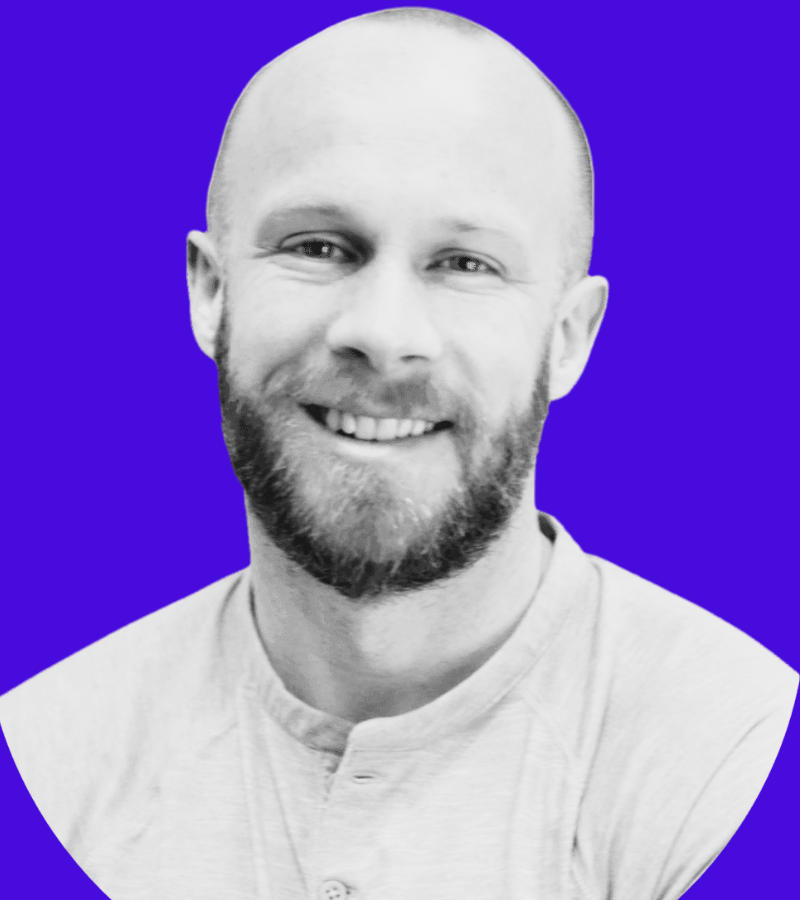
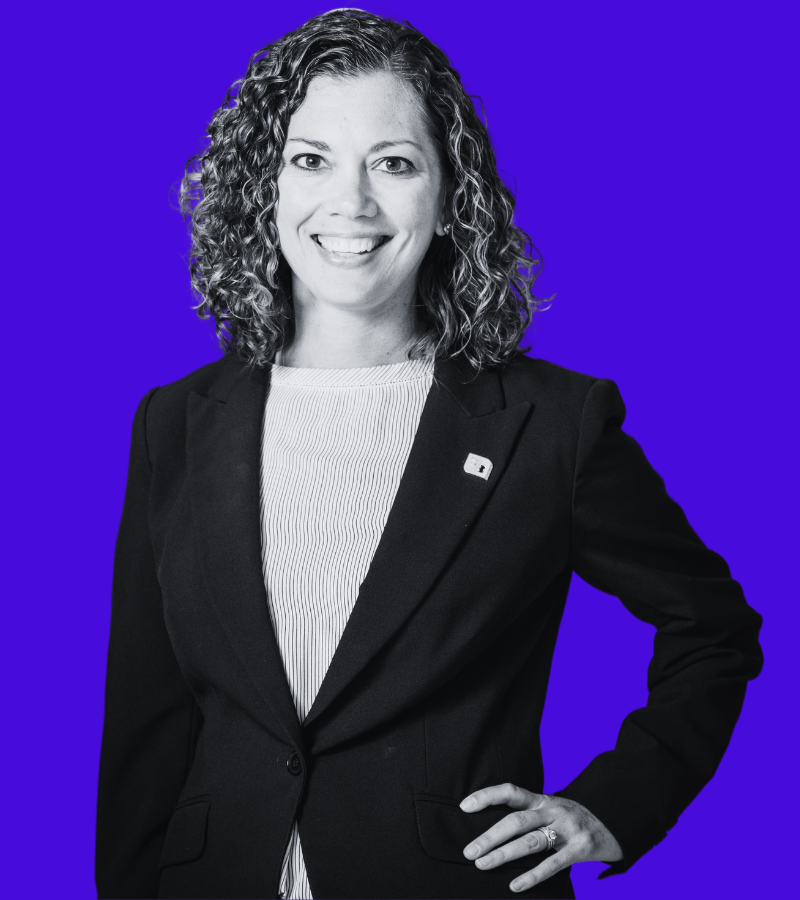
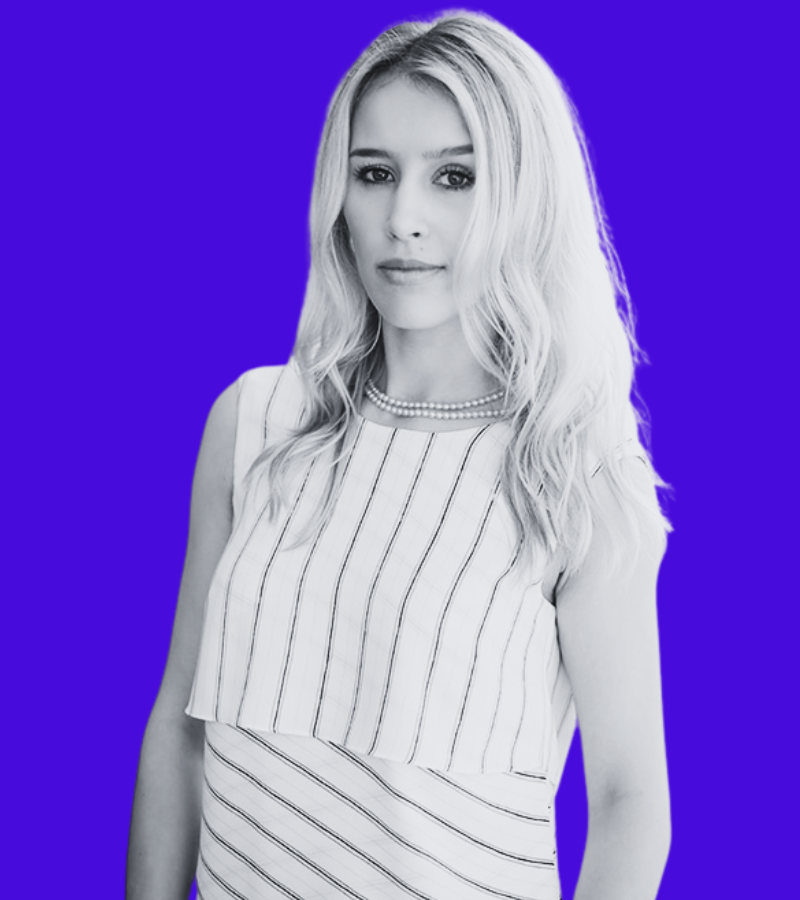
1. How Amex is rebuilding its AI brain and the science of better models
Some financial institutions rush to bolt generative interfaces onto legacy systems, but Amex is taking a slower, deeper route. It’s working under the hood, reengineering the very tools that decide who gets approved, flagged, or rewarded — and how.
At the heart of this effort is a unit called Frontier Research, a relatively new addition to the company’s Global Decision Science (GDS) team. “We were officially formed last year to support a longer-term research and exploration agenda,” said Anton Hinel, VP of Frontier AI Research and Customer Insights at American Express. “The idea behind Frontier Research is to give researchers time and space to work on more long-term research — something that is not always possible for a conventional modelling team that owns and maintains a production model.”
While most data science teams are pulled toward business deadlines and production fires, the Frontier Research team operates more like a skunkworks lab — autonomous, exploratory, and deliberately unshackled from short-term metrics. Their goal isn’t just to iterate on what already works. The mandate is to go deep, not just fast.
The day-to-day operations of Global Decision Science teams are focused on maintaining and optimizing these production models. The Frontier Research team takes a step back and asks harder questions – ones that could influence the entire architecture of how American Express makes risk decisions. It’s not just how to improve a model, but whether there’s a better paradigm altogether.
“Our goal is to ask the tough questions that hopefully yield answers that apply to the entire company,” Hinel said.
Their research spans the full Amex customer journey from acquisition to long-term retention. But the real constraint isn’t scope, it’s scalability. Techniques that don’t apply broadly across the business quickly lose priority. The focus isn’t on chasing the newest trend — it’s about building breakthroughs that compound.
“Our process around prioritizing our research ideas is: we focus on trying to resolve problems that are scalable, spanning multiple use-cases and modelling applications,” said Hinel. “This way, if research turns out to be successful, we can make an impact on the business.”
2. Grasshopper Bank’s Mantra: Deliberate progress over rapid leaps
Grasshopper’s strategy is a model of intentional AI adoption. They aren’t rushing to automate everything but are focusing on areas where AI can have a real, tangible impact without displacing human judgment. It’s a reminder that AI doesn’t have to be a quick fix or a force of change; it can be a complementary one.
By focusing on productivity, automating simple tasks, and keeping humans in the loop, Grasshopper is positioning itself to scale efficiently while staying true to its human-centric values.
This type of back-office automation might sound mundane, but it’s a smart, foundational move. Reducing the amount of time spent on repetitive work unlocks a more engaged workforce focused on solving problems that AI can’t, yet.
Pete Chapman, the company’s CTO, sums up the firm’s philosophy toward this new technology: “AI means different things to different people,” emphasizing their careful approach to adopting AI where it makes sense.
In highly regulated areas like lending and due diligence, Grasshopper uses AI selectively to assist human decision-makers. Chapman stresses that AI recommendations will never replace human judgment in these areas. For example, while AI might assist in portfolio monitoring or due diligence tasks, the final decision on credit approval is always human.
The firm is also cautious about using generative AI in areas like risk and credit decisioning. In Chapman’s words, “Modern generative models often function as ‘black boxes’”, and that lack of transparency could undermine trust in the process. Choosing this route keeps both auditability and explainability front and center for the bank.
By mid-year, the bank plans to roll out more intelligent AI agents to handle complex tasks, which require nuance and critical thinking beyond what basic bots can handle. It’s about building scalable AI solutions that grow with the company’s evolving needs.
3. Fifth Third: AI at the intersection of tradition and innovation
At Fifth Third Bank, its AI-powered chatbot, Jeanie’s story didn’t start with strategy decks. It started with Covid-19 chaos and call centers overwhelmed by panicked customers. The bank isn’t just focusing on pushing the boundaries of AI technology; it’s also rebuilding its internal infrastructure to make it work.
“We were piloting the concept of a chatbot before Covid started, around 2019-2020,” recalled Michelle Grimm, Senior Director of Conversational AI at Fifth Third. “We started exploring the opportunity of how we could use this from a customer service standpoint. Then Covid happened, and we realized we had to quickly help our call center, which was overwhelmed with phone calls.”
But Jeanie’s story didn’t stop at crisis response, there’s more to it. Over the past few years, Grimm’s team at Fifth Third has transformed the bot from a rule-based responder into a nuanced intent recognizer, lifting NLU accuracy from 20% to 87%. “We utilized a more complex nested structure from an NLU perspective, and the model continues to evolve further,” she said.
The team’s approach is methodical. Train, test, monitor, refine — that’s the cycle. “The process is definitely rigorous,” when it comes to testing Jeanie, Grimm shared.
Akin to Grasshopper’s strategy, Fifth Third has so far steered clear of using generative AI for Jeanie – but never say never. “At this time, we are not using generative AI, but that doesn’t mean in the future we won’t explore it as that technology evolves,” she explained. Additionally, agentic AI, task automation, multilingual support, and even voice interaction are on the company’s roadmap — but only when the customer value and operational readiness align.
Fifth Third’s differentiation in Jeanie’s inner workings lies in rigor and disciplined execution. Every flow is tested by quality engineers, fine-tuned according to user feedback, and monitored post-deployment. “Even after going live, we continuously monitor and refine the chatbot to ensure it meets customer expectations. We have multiple steps from development to production and post-production evaluation to improve the experience,” Grimm said.
4. Credit Karma frames GenAI as a helpful partner in making AI more understandable
Credit Karma is building with generative AI as a trust-building layer on top of its traditional Recommendations Engine. The firm is also using generative AI to create personalized, context-driven content that explains shifts in credit scores, loan options, and financial products in ways that users can easily understand.
According to Maddie Daianu, Head of Data and AI at Credit Karma, the idea of expanding GenAI capabilities with an embedded approach emerged from identifying a clear gap. The firm found that despite significant advancements in using data, machine learning, and AI to help members understand and manage their finances, many US consumers still lack the confidence to make sound financial decisions.
One of the ways Credit Karma is using GenAI is through Intuit Assist. This GenAI-powered financial assistant provides financial guidance and capabilities across the company’s product ecosystem, including TurboTax, QuickBooks, and Mailchimp. Then there’s the ‘See Why’ feature powered by Intuit Assist, which explains credit card and loan suggestions in context. It’s part of a broader integration with Intuit’s GenOS infrastructure, which allows Daianu’s team to embed AI insights directly into the Credit Karma user interface, not as a separate assistant, but as a narrator inside the journey.
What makes Credit Karma’s approach strong is how traditional ML and GenAI complement each other: the former makes the right offer at the right time, the latter explains why. This distinct approach to applying generative AI also serves as a strategic differentiator for the company.
Credit Karma’s Recommendations Engine is built on its advanced machine learning infrastructure, which ranks content and offers based on each member’s individual financial profile. The recommendations come from ML, but GenAI powers the ‘See Why’ feature to make those choices more understandable.
“While our recommendations engine intelligently serves members relevant content and offers, Intuit Assist helps contextualize to the member as to why we suggest a certain insight or offer,” said Daianu.
Credit Karma’s approach is about building consumer trust through transparency. The ‘why’ behind a recommendation is just as important as the recommendation itself, Daianu explained.
Four Paths, One Core Principle: AI must serve, not shine
These four companies — a legacy card issuer, a digital SMB bank, a regional institution, and a consumer fintech — differ in their AI infrastructure, ambition, and velocity. But their underlying themes are largely aligned, and the lessons go deeper:
- AI must be trustworthy before it can be transformational: From Hinel’s research at Amex to Grimm’s rigorous testing at Fifth Third, every executive emphasized the primacy of building trust in financial AI.
- Personalization drives loyalty: Fifth Third and Credit Karma are tailoring the customer experience, with AI providing personalized advice, recommendations, and insights. These companies understand that AI can enhance relationships with customers by making each interaction feel relevant.
- Explainability is the new UX, and transparency builds trust: Credit Karma and Grasshopper’s approach shows how customers want understanding, not just automation. Whether through contextualized GenAI or simple nudges, transparency drives engagement and can demystify complex financial decisions.
- Feedback, in addition to data, trains good AI: Fifth Third’s post-call reviews and Jeanie refinements, or Credit Karma’s behavioral loop between interaction and prediction, demonstrate how human insight still shapes machine intelligence.
- You don’t have to chase GenAI — but you better understand it: Each company is calibrating its use of generative AI based on its maturity, use case, and customer trust levels. The shared reality is that, hype aside, none of them are actually adopting it blindly.
There’s also another clear thread running through these stories — AI isn’t about replacing humans, it’s about augmenting human decision-making to improve the customer experience. Whether that means enhancing personalized recommendations or automating tedious tasks, AI is being deployed in diverse ways across these companies to create value for end customers eventually.
These narratives also shed light on the fact that building good AI is about facing the boring, difficult, everyday work of getting it right. That means clean data, explainable outputs, interdisciplinary teams, internal patience, and constant feedback.
As Daianu pointed out, deploying GenAI internally requires a diverse team of specialists — data scientists, engineers, and ML experts — working to tailor the tech to specific products. Building this capability takes significant time and investment. It’s not an overnight transformation, and without dedicated resources, implementations will fall short of their potential.